A00-240: SAS Statistical Business Analysis Using SAS 9: Regression and Modeling
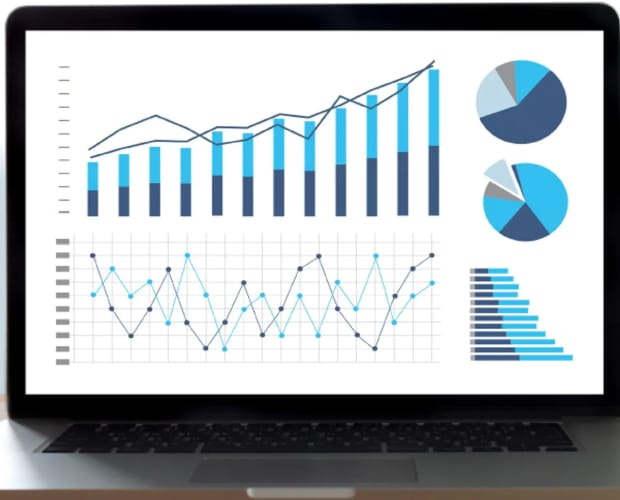
What’s included
$14.99 / $24.99
Get ready for your exam by enrolling in our comprehensive training course. This course includes a full set of instructional videos designed to equip you with in-depth knowledge essential for passing the certification exam with flying colors.
Pay once, own it forever
Video Courses
Free cloud-based SAS software option for learning: SAS OnDemand for Academics
Lectures | Duration |
---|---|
1. Create a SAS account to access SAS ondemand for Academics | 3m |
2. Upload course data files and SAS programs into SAS ondemand for academics | 6m |
3. change file path/directory in SAS ondemand for academics | 7m |
4. examples: update and run SAS programs in SAS ondemand for academics | 7m |
1. Create a SAS account to access SAS ondemand for Academics
3m
2. Upload course data files and SAS programs into SAS ondemand for academics
6m
3. change file path/directory in SAS ondemand for academics
7m
4. examples: update and run SAS programs in SAS ondemand for academics
7m
Analysis of Variance (ANOVA)
Lectures | Duration |
---|---|
1. ANOVA 0 | 10m |
2. Using Proc Univariate to Test the Normality Assumption Using the K-S Test | 3m |
3. ANOVA 1 | 10m |
4. ANOVA 2 | 7m |
5. ANOVA 3 | 4m |
6. ANOVA 4 | 4m |
7. ANOVA 5 | 3m |
8. ANOVA 6 | 4m |
9. ANOVA 7 | 12m |
10. ANOVA 8 | 10m |
11. ANOVA 9 | 16m |
12. ANOVA 10 | 3m |
13. ANOVA 11 | 3m |
14. ANOVA 12 | 5m |
15. ANOVA 13 | 8m |
16. ANOVA 14 | 11m |
17. ANOVA 15 | 3m |
18. ANOVA 16 | 3m |
1. ANOVA 0
10m
2. Using Proc Univariate to Test the Normality Assumption Using the K-S Test
3m
3. ANOVA 1
10m
4. ANOVA 2
7m
5. ANOVA 3
4m
6. ANOVA 4
4m
7. ANOVA 5
3m
8. ANOVA 6
4m
9. ANOVA 7
12m
10. ANOVA 8
10m
11. ANOVA 9
16m
12. ANOVA 10
3m
13. ANOVA 11
3m
14. ANOVA 12
5m
15. ANOVA 13
8m
16. ANOVA 14
11m
17. ANOVA 15
3m
18. ANOVA 16
3m
Prepare Inputs Vars for predictive Modeling
Lectures | Duration |
---|---|
1. Prepare Inputs Vars_1 | 6m |
2. Prepare Inputs Vars_2 | 13m |
3. Prepare Inputs Vars_3.Categorical Input Variable_1.Knowledge points | 5m |
4. Prepare Inputs Vars_3 | 7m |
8. Prepare Inputs Vars_4 | 11m |
10. Prepare Inputs Vars_5 | 5m |
1. Prepare Inputs Vars_1
6m
2. Prepare Inputs Vars_2
13m
3. Prepare Inputs Vars_3.Categorical Input Variable_1.Knowledge points
5m
4. Prepare Inputs Vars_3
7m
8. Prepare Inputs Vars_4
11m
10. Prepare Inputs Vars_5
5m
Linear Regression Analysis
Lectures | Duration |
---|---|
1. Exploring the Relationship between Two Continuous Variables using Scatter Plots | 10m |
2. Producing Correlation Coefficients Using the CORR Procedure | 15m |
3. Multiple Linear Regression: fit multiple regression with Proc REG | 10m |
4. Multiple Linear Regression: Measures of fit | 6m |
5. Multiple Linear Regression: Quantifying the Relative Impact of a Predictor | 3m |
6. Multiple Linear Regression: Check Collinearity Using VIF, COLLIN, and COLLINOINT | 11m |
7. fit simple linear regression with Proc GLM | 15m |
8. Multiple Linear Reg: Var Selection With Proc REG:all possible subset: adjust R2 | 12m |
9. Multiple Linear Reg: Var Selection With Proc REG:all possible subset: Mallows Cp | 6m |
10. Multiple Linear Regression:Variable Selection With Proc REG:Backward Elimination | 8m |
11. Multiple Linear Regression:Variable Selection With Proc REG: Forward selection | 9m |
12. Multiple Linear Regression:Variable Selection With Proc REG: Stepwise selection | 4m |
13. Multiple Linear Regression:Variable Selection With Proc GLMSELECT | 15m |
14. Multiple Linear Regression: PowerPoint Slides on regression assumptions | 8m |
15. Multiple Linear Regression: regression assumptions | 13m |
16. Multiple Linear Regression: PowerPoint Slides on influential observations | 11m |
17. Multiple Linear Regression: Using statistics to identify influential observation | 18m |
1. Exploring the Relationship between Two Continuous Variables using Scatter Plots
10m
2. Producing Correlation Coefficients Using the CORR Procedure
15m
3. Multiple Linear Regression: fit multiple regression with Proc REG
10m
4. Multiple Linear Regression: Measures of fit
6m
5. Multiple Linear Regression: Quantifying the Relative Impact of a Predictor
3m
6. Multiple Linear Regression: Check Collinearity Using VIF, COLLIN, and COLLINOINT
11m
7. fit simple linear regression with Proc GLM
15m
8. Multiple Linear Reg: Var Selection With Proc REG:all possible subset: adjust R2
12m
9. Multiple Linear Reg: Var Selection With Proc REG:all possible subset: Mallows Cp
6m
10. Multiple Linear Regression:Variable Selection With Proc REG:Backward Elimination
8m
11. Multiple Linear Regression:Variable Selection With Proc REG: Forward selection
9m
12. Multiple Linear Regression:Variable Selection With Proc REG: Stepwise selection
4m
13. Multiple Linear Regression:Variable Selection With Proc GLMSELECT
15m
14. Multiple Linear Regression: PowerPoint Slides on regression assumptions
8m
15. Multiple Linear Regression: regression assumptions
13m
16. Multiple Linear Regression: PowerPoint Slides on influential observations
11m
17. Multiple Linear Regression: Using statistics to identify influential observation
18m
Logistic Regression Analysis
Lectures | Duration |
---|---|
1. Logistic Regression Analysis: Overview | 10m |
2. logistic regression with a continuous numeric predictor Part 1 | 5m |
3. logistic regression with a continuous numeric predictor Part 2 | 15m |
4. Plots for Probabilities of an Event | 5m |
5. Plots of the Odds Ratio | 6m |
6. logistic regression with a categorical predictor: Effect Coding Parameterization | 10m |
7. logistic reg with categorical predictor: Reference Cell Coding Parameterization | 5m |
8. Multiple Logistic Regression: full model SELECTION=NONE | 8m |
9. Multiple Logistic Regression: Backward Elimination | 8m |
10. Multiple Logistic Regression: Forward Selection | 6m |
11. Multiple Logistic Regression: Stepwise Selection | 7m |
12. Multiple Logistic Regression: Customized Options | 12m |
13. Multiple Logistic Regression: Best Subset Selection | 5m |
14. Multiple Logistic Regression: model interaction | 14m |
15. Multiple Logistic Reg: Scoring New Data: SCORE Statement with PROC LOGISTIC | 6m |
16. Multiple Logistic Reg: Scoring New Data: Using the PLM Procedure | 5m |
17. Multiple Logistic Reg: Scoring New Data: the CODE Statement within PROC LOGISTIC | 4m |
18. Multiple Logistic Reg: Score New Data: OUTMODEL & INMODEL Options with Logistic | 5m |
1. Logistic Regression Analysis: Overview
10m
2. logistic regression with a continuous numeric predictor Part 1
5m
3. logistic regression with a continuous numeric predictor Part 2
15m
4. Plots for Probabilities of an Event
5m
5. Plots of the Odds Ratio
6m
6. logistic regression with a categorical predictor: Effect Coding Parameterization
10m
7. logistic reg with categorical predictor: Reference Cell Coding Parameterization
5m
8. Multiple Logistic Regression: full model SELECTION=NONE
8m
9. Multiple Logistic Regression: Backward Elimination
8m
10. Multiple Logistic Regression: Forward Selection
6m
11. Multiple Logistic Regression: Stepwise Selection
7m
12. Multiple Logistic Regression: Customized Options
12m
13. Multiple Logistic Regression: Best Subset Selection
5m
14. Multiple Logistic Regression: model interaction
14m
15. Multiple Logistic Reg: Scoring New Data: SCORE Statement with PROC LOGISTIC
6m
16. Multiple Logistic Reg: Scoring New Data: Using the PLM Procedure
5m
17. Multiple Logistic Reg: Scoring New Data: the CODE Statement within PROC LOGISTIC
4m
18. Multiple Logistic Reg: Score New Data: OUTMODEL & INMODEL Options with Logistic
5m
Measure of Model Performance
Lectures | Duration |
---|---|
1. Measure of Model Performance: Overview | 10m |
2. PROC SURVEYSELECT for Creating Training and Validation Data Sets | 10m |
3. Measures of Performance Using the Classification Table: PowerPoint Presentation | 7m |
4. Using The CTABLE Option in Proc Logistic for Producing Classification Results | 10m |
5. Assessing the Performance & Generalizability of a Classifier: PowerPoint slides | 4m |
6. The Effect of Cutoff Values on Sensitivity and Specificity Estimates | 11m |
7. Measure of Performance Using the Receiver-Operator-Characteristic (ROC) Curve | 7m |
8. Model Comparison Using the ROC and ROCCONTRAST Statements | 5m |
9. Measures of Performance Using the Gains Charts | 11m |
10. Measures of Performance Using the Lift Charts | 4m |
11. Adjust for Oversample: PEVENT Option for Priors & Manually adjust Classification | 16m |
12. Manually Adjusting Posterior Probabilities to Account for Oversampling | 5m |
13. Manually Adjusted Intercept Using the Offset to account for oversampling | 7m |
14. Automatically Adjusted Posterior Probabilities to Account for Oversampling | 6m |
15. Decision Theory: Decision Cutoffs and Expected Profits for Model Selection | 12m |
16. Decision Theory: Using Estimated Posterior Probabilities to Determine Cutoffs | 5m |
1. Measure of Model Performance: Overview
10m
2. PROC SURVEYSELECT for Creating Training and Validation Data Sets
10m
3. Measures of Performance Using the Classification Table: PowerPoint Presentation
7m
4. Using The CTABLE Option in Proc Logistic for Producing Classification Results
10m
5. Assessing the Performance & Generalizability of a Classifier: PowerPoint slides
4m
6. The Effect of Cutoff Values on Sensitivity and Specificity Estimates
11m
7. Measure of Performance Using the Receiver-Operator-Characteristic (ROC) Curve
7m
8. Model Comparison Using the ROC and ROCCONTRAST Statements
5m
9. Measures of Performance Using the Gains Charts
11m
10. Measures of Performance Using the Lift Charts
4m
11. Adjust for Oversample: PEVENT Option for Priors & Manually adjust Classification
16m
12. Manually Adjusting Posterior Probabilities to Account for Oversampling
5m
13. Manually Adjusted Intercept Using the Offset to account for oversampling
7m
14. Automatically Adjusted Posterior Probabilities to Account for Oversampling
6m
15. Decision Theory: Decision Cutoffs and Expected Profits for Model Selection
12m
16. Decision Theory: Using Estimated Posterior Probabilities to Determine Cutoffs
5m